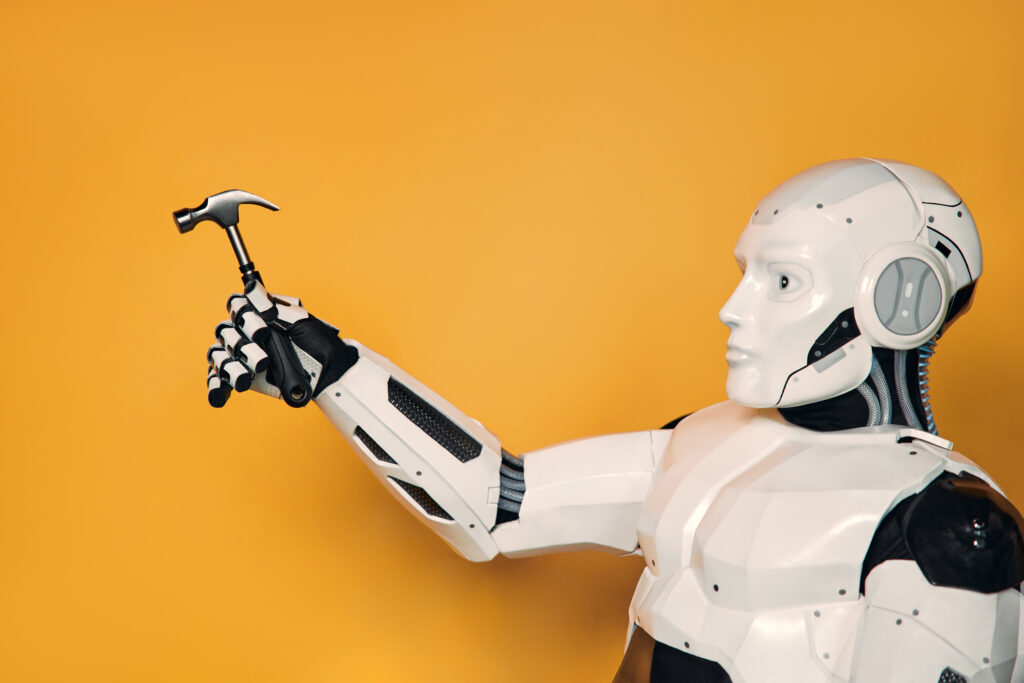
We often hear talk of PoC Purgatory or the PoC Loop — where people get stuck producing Proofs of Concept, failing to progress in their Artificial Intelligence (AI) journey, and failing to realise the benefits they were expecting. In this article, I will look at some ways to address these problems.
Choose the Right Name
As with so many fields, when it comes to AI, terminology matters. One term that has been widely used but often misunderstood is PoC. There is nothing wrong with the term, but it is intended to Prove (as in test) whether a Concept (an idea) is feasible, usually from a technical perspective. When it comes to AI, most of the popular use cases/solutions have already been proven. So you only really need to Prove a Concept when you’re at the leading edge of technology.
This matters because the wrong name can point you in the wrong direction. So all too often people get to the end of the PoC, and the team thinks they’ve succeeded (they possibly have, technically), but everyone else is underwhelmed or they are impressed by the technology but don’t see where the business value will come from.
So I recommend two alternative approaches:
- Proof of Value (PoV)
- Proof of Context (I don’t have a good abbreviation)
In my experience, these are more appropriate and meaningful terms. Here’s why:
Focus on Value Over Feasibility
Talking PoV shifts the focus from merely proving that something can be done to proving that it should be done because it adds value. In fact, I would recommend at least drafting your AI business case before starting a PoV. If you don’t know where the value is coming from, what are you trying to prove?
Ideally get business case approval, at least at the high level before proceeding with your PoV. This helps ensure you are delivering what senior stakeholders consider suitable, acceptable and sufficiently valuable. All the usual caveats for high-level / early work — use suitably wide ranges of value for both costs and benefits. If you go with a single number, that will stick in people’s minds and they are more likely to try and hold you to that number in the future.
Remember the People
Ensure you look at people change from the outset. It is almost certain that AI is going to change the way people work. Will they welcome it? e.g. it is taking a load of boring paperwork off their plates, stuff they often do at home and resent. Or will they feel threatened, that their professional competence is being called into question, that job cuts are coming?
AI is no different from other technology projects. No matter how good the technical output, if the people aren’t on board you won’t achieve the outcome and realise the value. This will make your job even harder when it comes to getting the next phase or next project off the ground.
“Go Home Early” Benefits won’t be Enough
Spend time getting the business case right. All too often I see people taking the easy option. “AI will save 1,000 hours for 50 people every month and given the average salary of £xx,000 we will save £yy,000”. That might look good in Excel, but what if you get challenged — “where can I find these numbers in the budget / P&L / Balance Sheet?” This is then countered by “They will be freed up to do more valuable work”. A noble intention, but unless you can identify and quantify the other work you’re probably just letting people go home early.
In some cases this can work — e.g. if attrition of staff is a significant issue, with the burden of paperwork in the evenings and weekends being a major contributor to people leaving, then you could be on to something. Then you need to quantify the cost of staff replacement — temporary cover, hiring fees, training time and costs, loss of repeat business, loss of customers etc. This can make a compelling case with even a small percentage reduction in attrition, with a project that staff will be very excited about.
Focus on Your Context and Specific Challenges
This is where the Proof of Context comes into play. A PoC (Proof of Concept) might demonstrate that an AI model works in a controlled environment, but that rarely translates into real-world success. A Proof of Context, on the other hand, involves testing the AI solution in your real-world setting, ensuring it delivers value under your actual operating conditions. This helps identify potential issues early and ensures the solution is achievable and scalable.
For example, if you are considering using AI to work on uploaded documents or recorded conversations the technology is currently very good at doing this, nothing to prove here. But what if your business relies on lots of hand-written faxes that are scanned in (you’d be surprised at how often this still happens). What if there are lots of “unwritten rules” on how these faxes are written and interpreted or lots of formatting variations. These are your Context and you should be checking that you can get the results you need. What if you are in the medical field and have prescriptions written by doctors (with notoriously illegible handwriting) arriving by fax, but you need these to be processed into a very standardised format for legal compliance, good health outcomes and (if you’re not using the NHS) insurance applications. In this case you have a complex Context and it’s worth spending the time on proving it.
Stakeholder Engagement
Engaging stakeholders is crucial for the success of any AI project. You need to involve stakeholders from the outset, focusing on their needs and expectations. By demonstrating Value and Context early on, you build trust and foster collaboration, which is essential for the long-term success of the project.
Iterative Improvement
Finally, don’t forget that AI projects benefit from an iterative approach, where solutions are continuously refined based on feedback and performance. These approaches support this by focusing on delivering incremental Value in relevant Contexts. Each iteration provides an opportunity to learn, adjust, and improve, ensuring the evolving solution delivers the expected value.
Conclusion
In the context of AI, Proof of Concept falls short of addressing the critical need to demonstrate tangible value that can be delivered in your organisation. Proof of Value and Proof of Context are more fitting terms that align with business objectives, emphasise real-world application, and foster stakeholder engagement. By adopting a Value and Context approach, underpinned by a sound business case you have the best chance of delivering the AI-enabled outcomes you were hoping for.